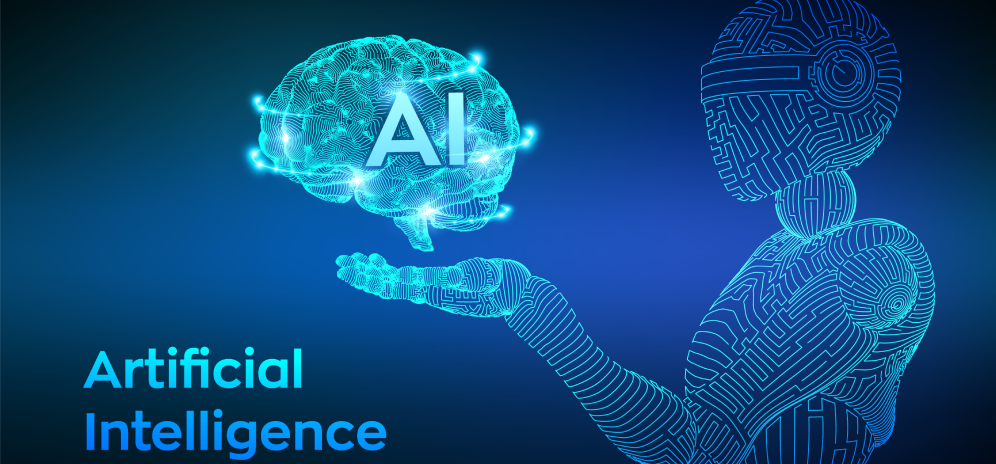
In recent years, the rapid advancements in Artificial Intelligence (AI) and machine learning have transformed numerous industries and revolutionized the way we interact with technology. However, as AI becomes increasingly complex and pervasive, the need for transparency and understanding of its decision-making processes has emerged. This is where Explainable AI (XAI) comes into play. In this blog post, we will delve into the concept of Explainable AI, its significance, and how it helps us interpret and comprehend machine learning models.
Understanding the Need for Explainable AI
As machine learning models become more sophisticated, they often operate as “black boxes,” making accurate predictions but lacking transparency in the decision-making process. This lack of interpretability raises concerns, particularly in critical domains such as healthcare, finance, and justice, where decisions have significant impacts on individuals’ lives. Explainable AI aims to address this challenge by providing human-understandable explanations for the outputs generated by AI models.
The Importance of Interpreting Machine Learning Models:
- Trust and Ethical Considerations: Explainable AI fosters trust in AI systems by allowing users and stakeholders to understand why certain decisions are made. It helps identify biases, errors, or unjust outcomes, promoting ethical and responsible AI deployment.
- Compliance and Regulation: In industries governed by regulations, such as healthcare and finance, the ability to interpret and explain AI models is essential to comply with legal requirements and ensure fairness and accountability.
- Debugging and Improvement: Explainable AI aids in diagnosing issues and improving models. By understanding the decision process, data scientists and developers can identify flaws, biases, or areas for improvement, leading to enhanced performance and reliability.
Methods and Techniques for Explainable AI:
- Feature Importance: This technique identifies the most influential features that contribute to a model’s decision-making process. By ranking features based on their impact, it provides insights into the factors driving predictions.
- Rule-based Explanations: Rule-based approaches provide understandable rules or decision trees that mimic the behavior of the AI model. These rules can be examined and validated to gain insights into how the model arrives at specific predictions.
- Local Explanations: Local explanations focus on individual predictions, explaining why a particular decision was made for a given input. Techniques such as LIME (Local Interpretable Model-Agnostic Explanations) provide interpretable approximations of complex models at the instance level.
- Model Distillation: Model distillation involves training a simpler and more interpretable model to mimic the behavior of a complex model. This distilled model is easier to interpret and can provide insights into the decision-making process.
Real-world Applications and Impact:
- Healthcare: Explainable AI enables doctors to interpret AI-driven medical diagnoses and treatment recommendations. It provides insights into the factors influencing predictions, enhancing trust, and enabling better-informed decision-making.
- Finance: Explainable AI helps financial institutions interpret credit scoring models, fraud detection algorithms, and investment strategies. It ensures transparency, fairness, and compliance with regulations.
- Autonomous Vehicles: Interpretable AI is crucial in the context of self-driving cars. By explaining the decisions made by AI systems, it enables passengers and regulators to understand the rationale behind actions, improving safety and public acceptance.
Conclusion:
Explainable AI plays a pivotal role in bridging the gap between complex AI models and human understanding. It empowers users, regulators, and stakeholders to comprehend the decision-making process, identify biases, ensure fairness, and build trust in AI systems. As we continue to rely on AI in critical domains, the pursuit of explainability is crucial for ethical and responsible AI deployment. By embracing Explainable AI techniques and practices, we can unlock the true potential of AI while ensuring transparency, accountability, and fairness in our AI-driven world.
As AI technologies continue to evolve, so does the need for explainability. Researchers and practitioners are actively exploring new methods and techniques to enhance the interpretability of machine learning models. The development of standardized frameworks and guidelines for explainable AI is also gaining momentum.
It is important for organizations to prioritize the adoption of explainable AI, not only to comply with regulations but also to foster trust among users and stakeholders. By investing in transparency and interpretability, companies can build stronger relationships with their customers and demonstrate their commitment to responsible AI practices.
In conclusion, explainable AI is a crucial aspect of modern machine learning. It allows us to understand and interpret complex AI models, ensuring transparency, fairness, and accountability. By embracing explainability, we can unlock the full potential of AI while addressing concerns about bias, ethics, and the impact of AI on society. As we move forward, it is imperative for tech companies to prioritize explainable AI and promote a culture of responsible AI deployment. Together, we can shape a future where AI systems are not only powerful but also transparent and understandable to everyone.
Recent Comments